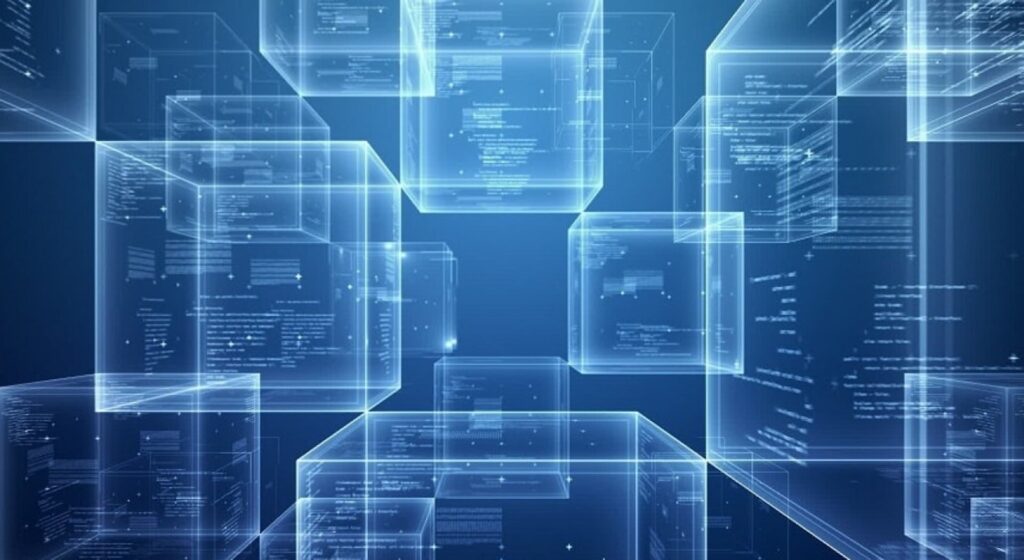
In the dynamic landscape of artificial intelligence and machine learning, reinforcement learning has emerged as a pivotal branch, offering a unique paradigm for training intelligent agents to make important decisions in complex and uncertain environments. Unlike traditional supervised or unsupervised learning, where algorithms rely on labeled or unlabeled data, reinforcement learning involves agents learning through interaction with an environment to maximize cumulative rewards.
This article delves into the realm of reinforcement learning, exploring its diverse real-world applications, spotlighting recent successes, and addressing the challenges that persist.
Real-world Applications of Reinforcement Learning
- Autonomous Systems: One of the most conspicuous domains where reinforcement learning is making significant strides in autonomous systems. The remarkable progress of self-driving cars serves as a prime example of how AI agents can navigate intricate road conditions, adhere to traffic rules, and adeptly evade obstacles. Similarly, drones utilize reinforcement learning to optimize flight trajectories, ensuring efficient and obstacle-free aerial missions.
- Robotics and Automation: Reinforcement learning is breathing new life into robotics by endowing machines with the capability to perform intricate tasks. In manufacturing, robots are being trained to manipulate objects, assemble products with precision, and even conduct quality checks. In healthcare, the surgical arena benefits from reinforcement learning, with robotic surgical systems collaborating seamlessly with surgeons to achieve unprecedented levels of precision in complex procedures.
- Gaming and Entertainment: The gaming industry has witnessed a remarkable transformation with the infusion of reinforcement learning. AI agents have not only achieved superhuman levels of performance in classic games like chess and Go but have also paved the way for new frontiers in entertainment. These AI agents can simulate human-level gameplay, ushering in innovative gaming experiences. In the realm of virtual reality, reinforcement learning contributes to the creation of immersive environments that respond dynamically to user interactions.
- Finance and Trading: The financial sector is leveraging reinforcement learning to optimize complex decision-making processes. Algorithmic trading, for instance, employs AI models to predict market trends, assess risk factors, and detect fraudulent activities. The integration of reinforcement learning into financial systems holds the potential to revolutionize investment strategies and risk management practices.
- Healthcare and Personalized Treatment: The healthcare sector is reaping the benefits of reinforcement learning, particularly in the domains of drug discovery and personalized treatment. AI agents are being trained to simulate molecular interactions, significantly expediting the drug discovery process. Additionally, reinforcement learning facilitates the creation of personalized treatment plans by predicting patient outcomes based on a multitude of variables.
Success Stories in Reinforcement Learning
- AlphaGo: Google’s DeepMind catapulted reinforcement learning to the forefront with the creation of AlphaGo, an AI program that achieved unparalleled mastery in the ancient game of Go. AlphaGo’s triumph over human world champions underscored the potential of reinforcement learning in tackling intricate challenges and mastering complex games.
- OpenAI’s Dactyl: OpenAI’s robotic hand, Dactyl, exemplifies the fusion of reinforcement learning and robotics. This innovative creation showcases the precision and dexterity that reinforcement learning can confer upon robotic systems. Dactyl’s ability to manipulate objects with remarkable finesse holds promise for applications in manufacturing and healthcare.
- Google’s DeepMind Energy Project: In a display of its real-world impact, DeepMind harnessed reinforcement learning to optimize energy consumption within Google’s data centers. The AI-driven energy project achieved a remarkable 40% reduction in energy usage, demonstrating the efficiency gains that AI-powered optimization can yield.
- Waymo: Google’s self-driving car subsidiary, Waymo, stands as a testament to the success of reinforcement learning in the realm of transportation. Through reinforcement learning, autonomous vehicles are trained to navigate complex and dynamic road scenarios, paving the way for safer and more efficient transportation solutions.
Challenges and Limitations
- Data Efficiency: One of the primary challenges associated with reinforcement learning lies in its data-intensive nature. Many reinforcement learning algorithms require a substantial number of interactions with the environment to learn effectively, which can be both time-consuming and resource-intensive.
- Reward Engineering: Designing appropriate reward functions is a complex task. The choice of rewards significantly impacts an agent’s behavior, and incorrect rewards can lead to suboptimal results or instability in learning.
- Generalization and Transfer Learning: Ensuring that agents can generalize their learned behaviors to new scenarios is a persistent challenge. Models trained in one environment may struggle to adapt to new situations, necessitating additional techniques for transfer learning.
- Safety and Ethics: As reinforcement learning is increasingly applied to real-world systems, ensuring safety and ethical behavior becomes critical. Erroneous policies learned by agents could lead to unintended and potentially harmful consequences.
Overcoming Challenges
In the dynamic landscape of reinforcement learning, innovation is a driving force that propels the field forward, aiming to conquer the challenges that it inherently presents. One of the noteworthy advancements in addressing these challenges is to enroll in Artificial Intelligence courses, and the second is the emergence of model-based reinforcement learning.
This approach seeks to alleviate the demand for an excessive number of real-world interactions, a characteristic associated with conventional reinforcement learning methods. Model-based reinforcement learning achieves this by constructing an internal model of the environment. This model serves as a simulation of the real-world environment, enabling agents to simulate interactions and learn from these simulated experiences.
By reducing the reliance on extensive real-world interactions, model-based reinforcement learning accelerates the learning process and conserves valuable resources.
Exploration strategies represent another innovative facet within the realm of reinforcement learning. The challenge of striking a balance between exploiting the learned knowledge and exploring new, uncharted territories is a fundamental one. Curiosity-driven learning offers a solution by encouraging agents to explore new states and actions, injecting an element of curiosity into their learning process. This mechanism is inspired by how humans learn – by seeking novel experiences and information.
Agents that utilize curiosity-driven learning actively seek out situations where their predictions or models of the environment are uncertain. This promotes the exploration of unexplored regions of the environment, leading to a more comprehensive and efficient learning process. The integration of curiosity-driven learning serves as a testament to how innovation in reinforcement learning can draw inspiration from human cognitive processes to enhance the capabilities of AI agents.
Meta-learning techniques present yet another stride in the ongoing innovation within the realm of reinforcement learning. These techniques capitalize on the concept of learning to learn. Meta-learning equips AI agents with the ability to rapidly adapt to new tasks by leveraging knowledge acquired from previous experiences.
Instead of starting from scratch with every new task, meta-learners glean insights from a library of past experiences, thereby accelerating the learning curve for novel tasks. This approach not only expedites the learning process but also fosters adaptability, enabling AI agents to handle a broader range of challenges with reduced data requirements.
In the intricate web of reinforcement learning, these innovations collectively represent the concerted efforts of researchers and practitioners to push the boundaries of what AI agents can achieve, effectively navigating the complexities and challenges inherent in their learning journeys.
Future Directions and Potential Impact
The fusion of reinforcement learning with other domains, like natural language processing and computer vision, promises to unlock new dimensions of AI-powered applications. Complex multi-agent systems stand to benefit from reinforcement learning, enhancing cooperation and coordination between agents.
In healthcare, reinforcement learning holds the potential to revolutionize diagnosis, treatment, and drug discovery, ushering in a new era of personalized medicine. Advancements in algorithms, computational architectures, and hardware will continue to push the boundaries of what reinforcement learning can achieve.
Conclusion
Reinforcement learning stands as a cornerstone of AI advancement, with a myriad of real-world applications shaping industries and driving innovation. The success stories of AI-powered systems like AlphaGo and autonomous vehicles underscore the transformative potential of reinforcement learning.
However, you will be able to understand the domain of cloud computing and its advancements through the best PG Program in AI and Machine Learning. The journey is not without its challenges, ranging from data efficiency to ethical considerations. Yet, ongoing research, collaborations between academia and industry, and the determination of experts are collectively forging a path toward even more remarkable applications of reinforcement learning in the future.
You may also like:- Top 20 Cybersecurity Career Options
- Top 5 Tips to Prevent Online Scams
- Top 10 Platforms to Learn Cybersecurity
- Top 7 Commercial Linux Distributions
- Why Do I Need a Website?
- Various Python Libraries for developing RESTful APIs
- Top 7 NodeJS Frameworks You Need To Know
- How Buying Instagram Followers Can Help Businesses Soar
- How To Find Gaps In Your Cybersecurity And How To Address Them
- How to close the site from indexing using robots.txt